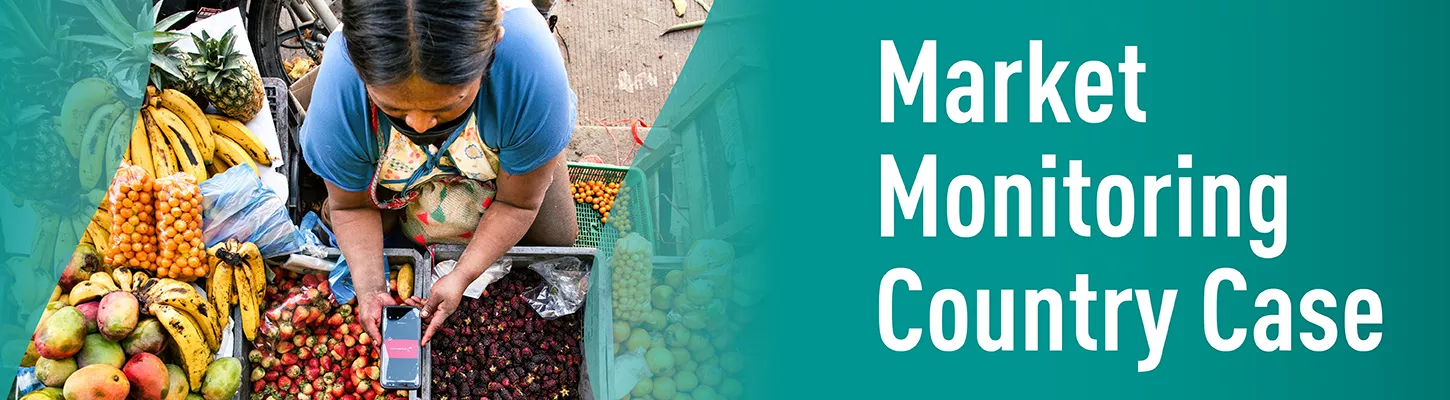
Research & Analysis
Publication
National Commission of the Retirement Savings System (Consar) of Mexico
Mexico’s National Commission of the Retirement Savings System (Consar) implemented a set of measures to produce high quality granular data reporting and invested in a machine learning tool as a substitute for manual data crunching. The result was an increased ability to identify patterns in the data and spot misconduct by regulated entities and their agents. For example, evidence of fraudulent account switching resulted in hundreds of agents being permanently banned from exercising any professional activity in the pension system. This action led to a reduction in fraud.
Type of market monitoring tool: Analysis of regulatory report
Sub-type: Analysis of granular data using suptech (supervisory technology)
Type of market monitoring tool: Analysis of regulatory report
Sub-type: Analysis of granular data using suptech (supervisory technology)
Background
FACTSHEET
Country: Mexico
Authority: Consar (National Commission of the Retirement Savings System)
Sector: Private pension fund administrators (“Afores”)
Tool: Suptech tool that uses machine learning to analyze large sets of granular data
What is the tool used for? To spot fraudulent, abusive, and anti-competitive behavior by Afores and their agents
Third parties: IDmission (vendor)
Estimated cost:
Year(s) of usage: Starting in 2016
*Does not include indirect costs, such as Consar staff time.
Country: Mexico
Authority: Consar (National Commission of the Retirement Savings System)
Sector: Private pension fund administrators (“Afores”)
Tool: Suptech tool that uses machine learning to analyze large sets of granular data
What is the tool used for? To spot fraudulent, abusive, and anti-competitive behavior by Afores and their agents
Third parties: IDmission (vendor)
Estimated cost:
*Does not include indirect costs, such as Consar staff time.
- Regulatory and supervisory powers. Consar is responsible for coordinating, regulating, supervising, and surveilling Mexico’s national pensions system, which is comprised of public pensions and the private system, operated by private pension administrators (collectively known as “Afores”). The system includes ten Afores and over 75,000 agents (locally known as “promoters”) servicing over 50 million pension account holders. Consar is autonomous and among other powers, holds the power to establish requirements on submission of information from Afores and to supervise them from a conduct and prudential perspective.
- Consumer protection supervision role. A conduct supervision unit does not exist as such. A centralized data intelligence team within the Operational Supervision Department is responsible for processing operational data collected from Afores and detecting behaviors that require further investigation by the department’s supervision team. A separate department focuses on financial supervision, including analyzing financial data for prudential purposes, with a focus on the safe financial management of pensioners’ funds. The data intelligence team engages with both the operational and financial supervision teams.
Purpose and incentives
- What is the tool used for? The tool allows Consar to analyze granular transaction data and to spot, essentially in real time, detrimental customer interactions with Afores, including fraudulent, abusive, and anticompetitive behaviors by these administrators and their agents. Customer interactions include account openings (for new customers), account closures, requests to switch to different Afores, withdrawals, and deposits.
- Incentives for tool development. Around 2013, Consar began working to address rampant fraud by Afores and their agents. Specifically, agents were switching pensioner accounts from one Afore to another and receiving commissions of approximately 2 percent on the account balance each time. The fraudulent activity was carried out by either “pushing” or deceiving customers into agreeing to a switch or by forging signatures. Switching could result in a loss for the customer since a destination Afore historically did not necessarily offer a higher return, better service, or lower fees. Also, due to the constant switching and low literacy rates, customers did not always know which Afore managed their savings or how much they had in their account. At the start of the project, Consar only possessed suspicions and consumer complaints about this type of malpractice, plus a diagnostic on the limitations of the retirement savings system’s nonfinancial operational framework.
- Data-driven supervisory process. Consar additionally wanted to investigate potential collusive or anticompetitive behavior among several Afores suspected of entering into an agreement to “not steal” each other’s client accounts. Consar needed to prove these suspicions but did not have the data. It decided to revamp the entire data collection system and introduce a data-driven supervisory process. To do so, it first mandated Afores to fully digitize client-facing processes so it could generate analyzable digital data from each client interaction. The data are stored in a central database at Procesar, a third-party company owned by the Afores. Procesar conducts reporting to Consar almost in real time (i.e., Consar downloads data from Procesar every two hours).
Technical methodology and data ecosystem
- Consar took several preliminary steps to implement the tool:
- Issued a 2014 regulation requiring the Afores to carry out digitized procedures and prohibiting them from using paper-based procedures for customer interactions. Interactions needed to be entirely completed on devices such as tablets that digitally connect to Afore information systems. In turn, each Afore is connected to Procesar (where data are stored).
- Defined the minimum information to be captured and recorded for each type of customer interaction as a substitute for previously nonstandardized paper forms, including geolocation and time stamps
- Standardized data terms and formats for customer interactions
- Eliminated the option for agents to use paper-based records
- Built a detailed agent database at Procesar. Each transaction must identify which agent conducted it. The agent database includes data on each agent, such as personal data, fingerprints, address, phone number, photo, age, etc.
- Given the history of fraud and customer abuse, in 2015 Consar standardized the manner in which account switching could be completed:
- The digital standardized form for account switching includes a recording of the customer’s voice reading or repeating a standard statement as determined by Consar, acknowledging their desire to switch Afores.
- Within the interaction, agents are required to provide customers with information on historical returns paid by both destination and origin Afores. If the destination Afore had historically paid lower returns than the origin Afore, the customer must specifically acknowledge this information in the recording.
- All customer interaction data are generated in an Afore’s system directly from an agent’s device (often a tablet with geolocation capabilities) and transmitted to Procesar for data storage. Although the Afores own Procesar, the data it stores and processes are, by law, Consar’s property. Data are transferred to Consar approximately every two hours.
- The data feed a risk dashboard built and used by the data intelligence team, which shows, among other details, a map of transactions occurring across the country almost in real time. The team built risk indicators and thresholds that trigger alerts to the supervision teams for follow-up. Each transaction can be “zoomed in” on to show every detail about it (everything recorded in the client interaction digital forms). Each transaction provides access to full information about the agent conducting the transaction. Agent Profile reports are built with data retrieved from the agent database in combination with the transaction database. Each agent is risk-scored according to a color-coded methodology developed by the data intelligence team. All risk score details can be zoomed in on.
- Initially the agent risk score, risk dashboard, heatmaps, and alerts used relatively manual background procedures such as data downloads and rules (e.g., alerts) control. Visualization was performed using Microsoft Excel.
- Given the difficulty of processing and analyzing a large volume of data (millions of transactions daily), Consar decided to invest in a data analytics tool. It engaged a data analytics company to automate the risk monitoring and warning system via a machine learning application.
- Using real data provided by Consar as well as previous risk assessments, the company employed a deep learning application to identify patterns that could point to Afore and agent misconduct. Since the data used to train the application were real data, the accuracy of the machine-generated alerts and scoring could be confirmed through human-based past scoring and assessment.
Staff, expertise, and other requirements
- The data intelligence team is comprised of five employees with expertise in data analytics and statistics; one was reassigned from another unit and the others were new external hires. These employees built the first Excel-based risk dashboard and engaged with the vendor, IDmission, to develop the machine learning tool. They are currently responsible for managing the data collection process, maintaining the tool, engaging with the vendor (as needed), and interpreting tool results—the basis for internal reporting to supervision teams.
- In terms of data storage, Consar did not need to invest in local infrastructure since the solution uses a cloud provider (AWS) for data storage and processing. The cloud architecture is highly scalable and comparatively less expensive than building similar capabilities in-house.
Vendor selection and cost
- It became clear that it was necessary to find a more powerful method for going through vast amounts of granular data to identify patterns that might indicate misbehavior by regulated entities. The machine learning model, including piloting, cost less than US$100,000. It took approximately six months to train the application, pilot the tool, and, finally, put it into full production. IDmission was selected as the vendor, mostly due to its ongoing relationship with Consar and the industry, which already used its digital identification and authentication services. There was no tender process nor consideration of different technological solutions to address Consar’s analytics needs.
Benefits and impact
- More efficient use of staff time. One immediate benefit was the considerably reduced time the data intelligence team spent retrieving and uploading data and updating the risk dashboard. The time freed up was allocated to less mechanical and more analytical tasks, such as interpreting the results of the machine learning tool to determine appropriate engagement with supervisory teams for further investigation.
- Improved ability to identify risks and accordingly strengthen regulation. Another benefit was how, over time, the algorithms started to identify new types of risky behavior previously not identified by the original manual analysis system. The new system was able to spot outliers and new patterns. A simple example of “risky agent behavior” that could have become a case of fraud involved noticing that one agent had conducted a transaction in the south of the country in the morning and another in the north just a few hours later. This type of behavior could not have been identified by data originating from the paper-based forms Afores used. With digitization of all customer transactions and use of the machine learning application, the practice of agents enlisting third parties (e.g., friends, subcontractors) to conduct a large number of transactions (to generate fees) could easily be identified—almost in real time. Identification of the practice led to a further change in the regulation that requires all customer interactions to record agent and customer biometrics at the time of transaction. Now, only agents who are registered and have biometric information (fingerprints) in the agent database can conduct transactions. A transaction will not go through if someone falsely poses as an agent. After gradually building a customer biometrics database (using biometric technology offered by the same vendor that provided the machine learning technology for customer transaction analysis), Consar intends to require transaction approval only if the customer’s biometrics match those in the Consar system.
- Better monitoring of anticompetitive behavior. The machine learning application was able to create a new method to identify potential anticompetitive behavior by Afores, such as agreements not to “steal” each other’s clients. Digital evidence like this would not have been possible with the previous manual system, even when using the same data or with a hundred Consar employees crunching data full time. The algorithms specifically detected a suspicious absence of account switching between a certain group of Afores—an indication of potential collusion. The findings triggered further investigation and, in coordination with the competition commission, resulted in the largest-ever fine imposed on financial institutions in Mexico (see here in Spanish).
- Reduction in incidences of fraud. The changes noted above (digitization of operational procedures at Afores, standardization and near real-time reporting of granular data, revamping the agent database, including customer voice recordings in transaction records, and capturing agent and customer biometrics) have allowed Consar not only to identify and act upon instances of suspected fraud, but to substantially reduce the amount of fraud and transactions with negative consumer outcomes (e.g., account switching to an Afore that pays lower returns). Consar believes that reduction in fraud and other deleterious practices is related to the recognition by Afores that Consar is now able to more effectively, efficiently, and quickly identify misbehavior. Hence, the probability of facing enforcement action is higher.
- More effective prosecution of fraudsters. Another result is the referral of agents to the public prosecutor based on fraudulent behavior the machine learning tool has spotted. For example, the tool highlighted transaction patterns which raised the suspicion that transaction points, such as commercial establishments where customers make withdrawals and deposits, were requiring customers to make a purchase as a condition to conducting a financial transaction in their pension account. Another case that showed evidence of fraudulent account switching also resulted in hundreds of agents being permanently banned from exercising any professional activity within the pension system.
Limitations and implementation challenges
- Legal and regulatory measures. Consar initially did not have adequate data to confirm its suspicion of fraudulent actions in the Afore system. It first needed to spend time and effort to make the regulatory changes necessary for implementing a new data collection system. Consar’s strong and broad-based legal powers were instrumental in taking regulatory measures to increase timeliness, quality, and granularity of the reported data, and to set up the centralized Procesar database for data storage and retrieval, essentially in real time. These legal powers allowed Consar to impose digitization of operational processes at Afores, which increased the visibility of agent actions and made it easier for Afores and Consar to identify and take action against misbehavior. Consar also benefited from the legal clarity on its ownership of data submitted by Afores.
- Availability of skilled data analysts/scientists. The main challenge of implementation was setting up a team of data analytics and statistics experts with a proactive, predictive digital supervision mentality. The new supervision model required a change in hiring and training practices to ensure that staff was willing and able to perform the new tasks.
- Seamless integration with existing systems. Other challenges included integrating new machine learning-powered analytics with historical analytics and data to ensure continuity in supervisory intelligence with respect to individual Afores and agents, and to ensure comparability of data whose format and sources had fundamentally changed. Another challenge was Consar staff being mandated by regulation to download data from Procesar, which takes substantial time since it is done several times daily. A seamless and automated process would be preferred, but in Mexico’s case this requires legal reform and technical adjustments.
- Ensuring confidence in and effective use of machine learning results. Another challenge has been securing a high level of confidence in machine learning results, which required many rounds of experimentation with the vendor and for Consar to share additional batches of sensitive real data with the vendor, increasing third-party risk. The next and most continual challenge is to interpret the results of the machine learning tool to trigger appropriate and timely supervisory action. The tool does not provide “supervisory solutions,” only input for analysis which is often difficult to interpret. Industry resistance to imposed changes was also significant, including legal suits against Consar that questioned both the changes to internal Afores procedures and supervisory actions based on data analytics (e.g., references to public prosecutors). Moreover, some effort will be required to educate judges in courts where digital evidence produced by the machine learning tool is used against Afores and agents.
Future plans for the tool
- There are no immediate plans to modify the machine learning tool in the future. Since the pension system can only conduct limited types of customer transactions, the tool is considered strong enough to cover existing data analytics. Moreover, the tool’s usefulness and power increase as it learns from new data over time.
Learnings
- Digitization of customer interactions with Afores was key to setting up an essentially real-time monitoring system that allowed misbehavior to be identified. If both the regulated sector and the regulator continued to use paper-based controls and procedures, it would be difficult to guarantee effective digital supervision based on vast volumes of granular data.
- Consar had to use its ample legal powers to impose digitization at regulated entities in order to ensure the quality of reported data.
- A machine learning tool does not eliminate the need for intensive human-based data analytics, which is essential to interpreting the tool’s results and triggering timely and appropriate supervisory action.